In the Marketing Tech and Advertising Tech industries, data infrastructure has undergone significant transformations to keep pace with the demands of big data analytics and real-time decision-making. The landscape is characterized by a blend of both cloud and on-premises solutions, each offering distinct advantages.
This post explores the basics of the data infrastructure landscape. If you’re looking for a comprehensive deep dive – don’t miss our Data Infrastructure Buyer’s Guide!
On-Premises vs. Cloud-Based Data Infrastructure
When choosing a data infrastructure, organizations need to consider whether to deploy it on-premises or in the cloud. Both options have their advantages and disadvantages, and the choice depends on factors like data security, scalability, cost, and IT expertise.
On-premises data infrastructure refers to deploying and managing data infrastructure within an organization’s own data centers. This provides full control over data security and compliance, as well as the ability to customize the infrastructure to specific requirements. However, on-premises infrastructure can be costly to set up and maintain, and it may require dedicated IT resources for management and troubleshooting.
Cloud-based data infrastructure, on the other hand, refers to deploying and managing data infrastructure on cloud platforms like AWS, GCP, or Microsoft Azure. Cloud-based infrastructure offers scalability, flexibility, and cost-effectiveness, as organizations can easily scale resources up or down based on their needs. It also eliminates the need for upfront hardware investments and reduces the burden of infrastructure management. However, organizations need to ensure data security and compliance when moving sensitive data to the cloud, and they may need to rely on third-party vendors for support and maintenance.
On-Premises Solutions
While cloud solutions are prevalent, some organizations still maintain on-premises data infrastructure for various reasons, including regulatory compliance or specific security considerations. On-premises databases, data warehouses, and Hadoop clusters are often optimized for high-throughput processing of big data.
In terms of data organization, on-premises solutions may utilize optimized columnar databases and in-memory processing for faster analytics. Organizations might employ tools like Apache Spark for distributed data processing, ensuring efficient handling of vast datasets. However, data skew, ongoing resource management issues, and difficulties with integration, combined with the sheer complexity of running big data jobs in Spark and Hadoop are leading many organizations to seek more modern alternatives.
Cloud Solutions
Data infrastructure refers to the underlying foundation that enables the storage, processing, and analysis of data. It encompasses various components, including databases, data warehouses, data lakes, and more. These elements work in tandem to provide organizations with a scalable and reliable infrastructure to leverage their data effectively.
Data Lake vs. Data Warehouse: What’s the Difference?
Data lakes and data warehouses are two popular approaches for storing and managing data, each with its own strengths and use cases. A data lake is a centralized repository that stores raw and unprocessed data in its native format. It offers flexibility and scalability, allowing organizations to store vast amounts of structured, semi-structured, and unstructured data. On the other hand, a data warehouse is a structured repository that stores data in a predefined schema. It is optimized for querying and analysis, making it ideal for business intelligence and reporting purposes.
The Rise of the Data Lakehouse: Combining the Best of Both Worlds
The data lakehouse is an emerging concept that combines the strengths of data lakes and data warehouses, aiming to address their limitations. It leverages the scalability and flexibility of data lakes while providing the structured querying capabilities of data warehouses. By bringing together the best of both worlds, the data lakehouse enables organizations to perform advanced analytics on diverse datasets without compromising on performance or data governance.
Vendors in Cloud Data Infrastructure
Many organizations in Marketing and Advertising Tech leverage cloud platforms such as AWS, Google Cloud, and Azure for their scalability, flexibility, and cost-effectiveness. In the cloud, data is often stored in distributed databases, data lakes, or data warehouses. Modern cloud data solutions like Aqfer, AWS Redshift, BigQuery, and Snowflake, among others, provide a scalable architecture capable of handling massive datasets efficiently.
These platforms enable organizations to organize data in highly optimized formats, leveraging columnar storage and compression techniques. Parquet and ORC (Optimized Row Columnar) file formats are popular for storing large volumes of data efficiently. Cloud-based solutions also facilitate parallel processing, allowing organizations to process billions of records in parallel and derive actionable insights in near real-time. Further, many vendors offer add-on services to the primary cloud computing platforms such as Google Cloud and AWS as they offer highly optimized services that extend the functionality and scalability of the underlying cloud platform’s capabilities.
Native Solutions
The major cloud vendors, such as Amazon Web Services (AWS) and Google Cloud Platform (GCP), offer their own data warehousing solutions. AWS Redshift and Google BigQuery are two popular choices in this space.
Extended Solutions
To make the most of their ever-growing volumes of data, many businesses are turning to dedicated big data management platforms like Aqfer, Snowflake, and Databricks. Though cloud giants like AWS and Google Cloud offer data services, solutions from other platforms can provide businesses with deeper capabilities optimized for marketing, analytics, and data science use cases. The added power of these data-focused platforms comes from performance-enhancing features like caching, indexing, and query optimization that simplify working with massive, complex datasets. By leveraging the stability and scalability of major cloud infrastructure along with robust data tools, businesses can efficiently collect, process, and, and extract insights from their expanding data stores. The combination of cloud provider and specialized data platform gives businesses the best of both worlds – enterprise-grade infrastructure and cutting-edge data management functionality.
Dive Deeper
Ready to get serious about upgrading your data infrastructure? Our comprehensive Data Infrastructure Buyer’s Guide shared everything you need to know about the landscape to help you make informed decisions. From important emerging trends to vendor analysis to key strategic considerations for your data infrastructure, this guide has you covered! Click here to download the guide, or simply fill out the form below.
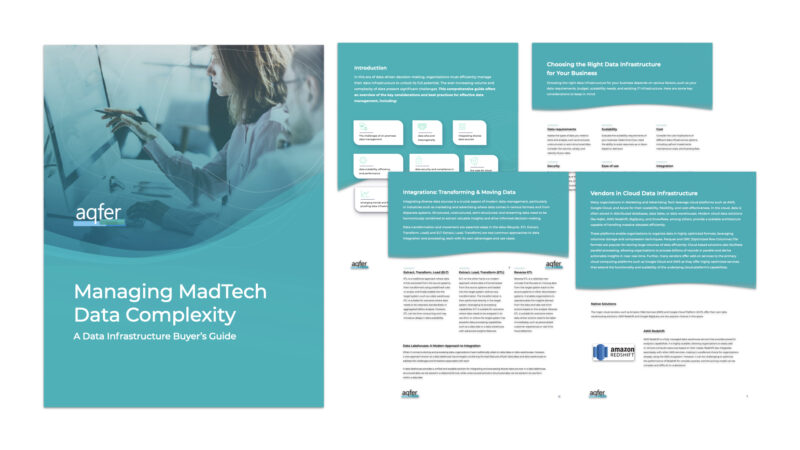