×
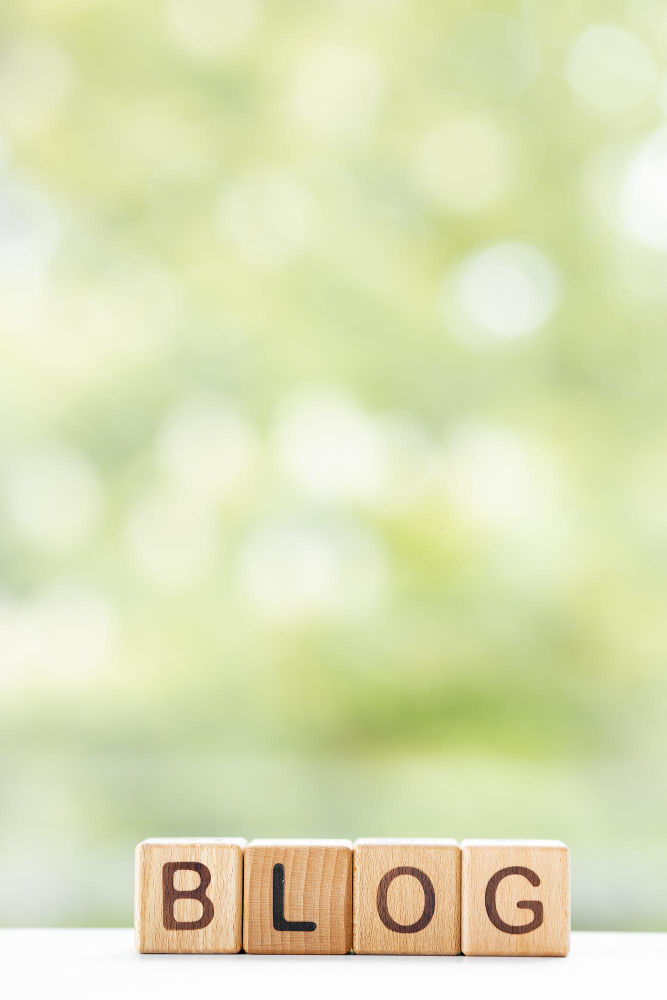
×
Aqfer Insights
Stay on top of the latest trends in Martech, Adtech, and Beyond
Subscribe to follow the news on what’s happening in the marketing data ecosystem.
In the realm of big data processing, the choice of tools and platforms can significantly impact both operational efficiency and financial outcomes. While solutions like Apache Spark have gained popularity for handling massive datasets, it’s crucial to understand its hidden costs and limitations. This post shares how Aqfer’s cost-effective and efficient solution for data processing at a massive scale.
Aqfer was developed to offer a more efficient and cost-effective approach to big data processing.
The advantages of Aqfer’s approach extend beyond immediate cost savings, offering long-term financial and operational benefits.
While Spark’s popularity is understandable given its processing capabilities, it’s essential to consider the total cost of ownership, including hidden expenses and operational inefficiencies. Aqfer offers a compelling alternative, designed to provide reliable, scalable, and cost-efficient data processing.
As data volumes continue to grow and the need for timely insights becomes increasingly critical, solutions like Aqfer that offer both performance and cost-effectiveness will be key to maintaining competitive advantage in data-driven industries. The future of big data processing lies not just in raw processing power, but in intelligent, efficient systems that maximize value while minimizing hidden costs. Click here to learn more about big data processing with Aqfer, or reach out for a tailored discussion about your organization’s current challenges and goals.
About the Author
Chief Executive Officer
Dan has provided strategic, tactical and technology advisory services to a wide range of marketing technology and big data companies. Clients have included Altiscale, ShareThis, Ghostery, OwnerIQ, Netezza, Akamai, and Tremor Media. Dan was the founder and CEO of Korrelate, a leading automotive marketing attribution company, purchased by J.D. Power in 2014. Dan is the former president of TACODA, bought by AOL in 2007, and was the founder and CTO of Permissus, an enterprise privacy compliance technology provider. He was the Founder and CTO of Engage and served as the acting CTO of CMGI. Prior to Engage, he was the director of High Performance Computing at Fidelity Investments and worked at Epsilon and Accenture (formerly Andersen Consulting).
Dan graduated magna cum laude with a BA in Astronomy and Astrophysics and Physics from Harvard University.