×
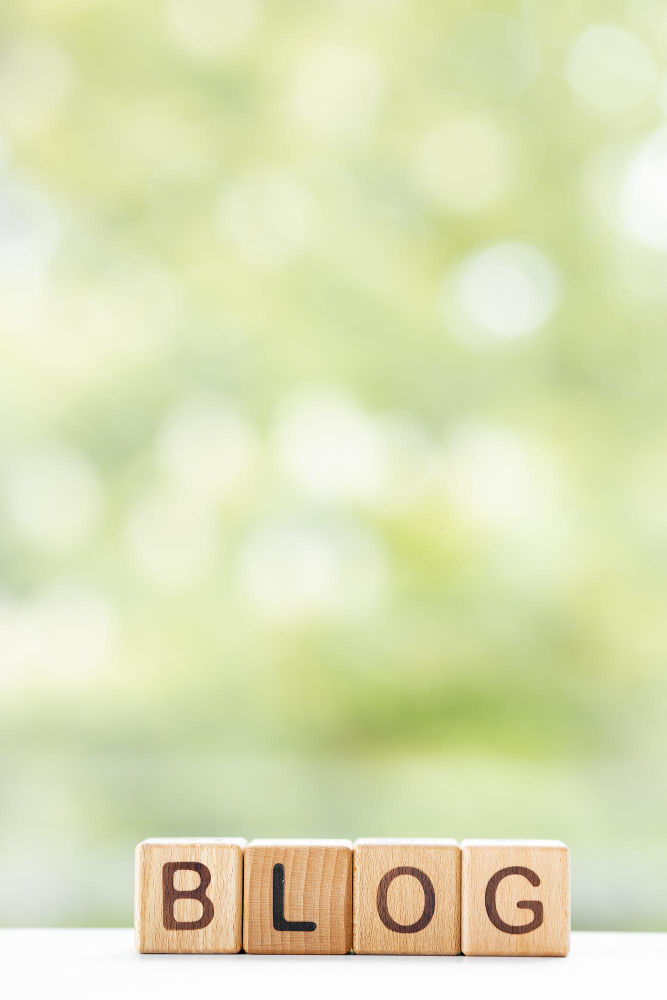
×
Aqfer Insights
Stay on top of the latest trends in Martech, Adtech, and Beyond
Subscribe to follow the news on what’s happening in the marketing data ecosystem.
For years, the tech industry has been abuzz with conversations around artificial intelligence (AI) and its transformative potential. As AI models become more sophisticated, the appetite for data to fuel them has grown exponentially. However, the traditional data retrieval methods have struggled to keep up with the ever-increasing demands of modern AI applications.
That is, until the advent of Retrieval Augmented Generation (RAG) – a game-changing innovation that is poised to revolutionize the way we think about data utilization in AI. As organizations seek to leverage their vast data assets more effectively, Retrieval Augmented Generation (RAG) bridges the gap between AI capabilities and real-world business applications. RAG represents a fundamental shift in how we access and leverage the wealth of data at our fingertips, unlocking new levels of efficiency, speed, and accuracy that were once unimaginable.
Retrieval Augmented Generation (RAG) is a cutting-edge method that enhances large language models by combining their language generation capabilities with real-time information retrieval. This approach allows AI models to deliver responses enriched with accurate and relevant details from trusted external data sources.
At its core, RAG relies on vector stores, which are databases optimized for similarity searches. These stores contain representations of data transformed into high-dimensional vectors using techniques like embeddings. When a user poses a query, the model converts the query into a vector and searches the vector store to find the most relevant pieces of data. These retrieved data chunks are then fed back to the language model as additional tokens to ground its response, ensuring the output is both contextually appropriate and factually reliable.
Advanced RAG applications can extend this concept by integrating structured data, APIs, and even real-time analytics into the retrieval process. For example, instead of relying solely on pre-embedded text, the system can dynamically query live databases or enterprise data lakes to retrieve the latest sales trends, customer insights, or campaign performance metrics. These integrations provide a richer context for generating actionable and accurate content, further enhancing the utility of RAG in specialized fields.
This combination of retrieval, generation, and advanced data integration minimizes the risk of inaccuracies (often referred to as “hallucinations”) and ensures the AI can handle complex, domain-specific tasks effectively.
At Aqfer, we’re making it seamless for businesses to use RAG technology in conjunction with our marketing data platform, enabling them to harness their data in powerful new ways. By pairing our robust data management capabilities with the benefits of advanced RAG implementations, we empower organizations to transform how they interact with and derive value from their data.
Our approach focuses on three key areas:
We’ve engineered our platform to seamlessly connect vast data repositories with RAG systems, ensuring that AI models can quickly access and utilize relevant information when generating responses. This results in more accurate, contextually appropriate outputs that directly reflect your organization’s specific knowledge and needs.
Through our advanced data processing capabilities, we enable RAG systems to access the most current information available, ensuring that AI-generated insights are always based on the latest data. This is crucial in the fast-paced world of marketing and advertising technology.
Our platform is designed to handle the growing demands of AI applications, allowing organizations to scale their RAG implementations without compromising on performance or accuracy. Whether you’re processing thousands or millions of data points, our infrastructure ensures smooth operation while being cost effective.
The practical applications are numerous – from generating detailed customer insights to powering intelligent chatbots and automating complex data analysis tasks. Our own internal chatbot, Aquabot, serves as a testament to the power of RAG technology when combined with our robust data infrastructure.
While implementing RAG technology, Aqfer is helping organizations:
As the appetite for data-driven insights continues to grow, the ability to effectively leverage that data has become a crucial competitive advantage. Retrieval AugmentedGeneration represents a pivotal shift in how we approach data utilization, empowering AI models to access the information they need with unprecedented speed, accuracy, and cost-efficiency.
By embracing the transformative power of RAG, businesses across industries can unlock new frontiers of innovation, drive better decision-making, and stay ahead of the curve in an increasingly data-driven world.
The future of AI lies in its ability to meaningfully interact with and utilize vast amounts of data, and when connecting RAG with Aqfer, that future is already here. Whether you’re looking to enhance your customer experience, streamline your operations, or gain deeper insights from your data, our RAG-enabled solutions can help you achieve your goals.
Ready to transform how your organization utilizes AI? Contact Aqfer today to learn how our RAG implementations can revolutionize your data