×
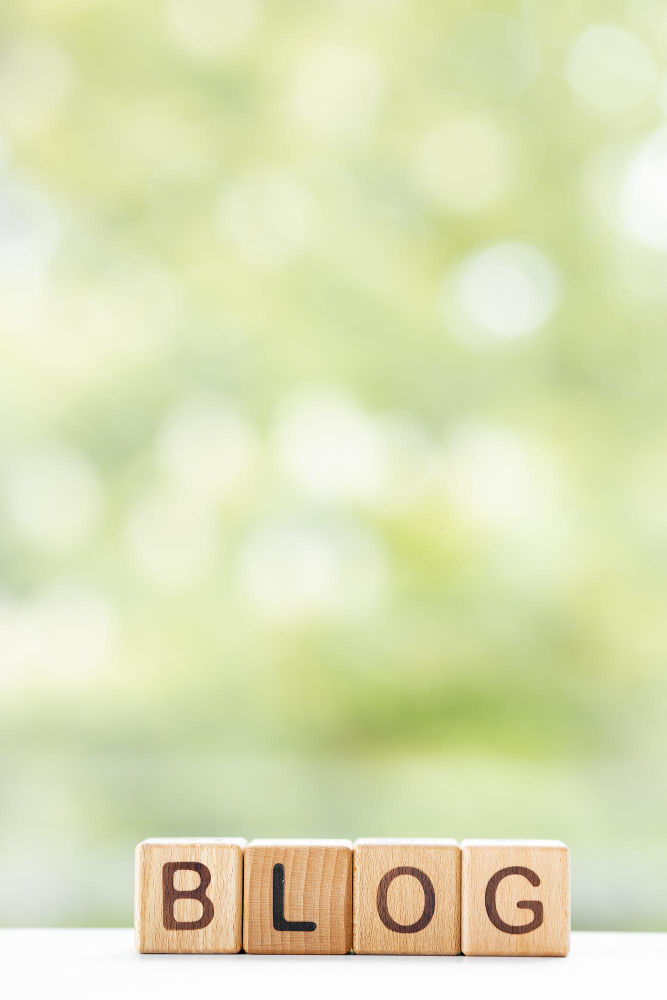
×
Aqfer Insights
Stay on top of the latest trends in Martech, Adtech, and Beyond
Subscribe to follow the news on what’s happening in the marketing data ecosystem.
Customer lifecycle management has undergone a dramatic transformation in recent years, driven by advancements in data analytics, artificial intelligence, and an increasing focus on personalized customer experiences. Traditionally, marketers relied heavily on segmentation strategies, grouping customers with similar characteristics to tailor their approaches. While this method has been a staple for decades, it’s increasingly falling short in today’s data-rich, hyper-personalized marketing landscape.
The limitations of traditional segmentation are becoming more apparent as customer expectations evolve. Broad demographic or behavioral categories often fail to capture the nuances of individual customer behavior and preferences. For instance, two customers in the same age group with similar purchase histories might have vastly different motivations, preferences, and potential lifetime values. Treating them identically based on their segment can lead to missed opportunities and suboptimal customer experiences.
This realization has paved the way for a new paradigm in customer lifecycle management: individual-level decision-making. Powered by advanced AI techniques like reinforcement learning, this approach, which we call “Next Best Everything,” moves beyond simple segmentation to treat each customer as a unique entity with their own evolving journey. Rather than applying predetermined rules based on segment membership, “Next Best Everything” continuously analyzes each customer’s behavior, context, and potential value to determine the optimal action at every touchpoint.
The “Next Best Everything” approach is built on principles that set it apart from traditional customer lifecycle management strategies:
It considers the entire customer lifecycle, from acquisition to retention and beyond, rather than focusing on isolated interactions or campaigns.
It coordinates decisions across all customer touchpoints, ensuring a consistent and optimized experience regardless of how or where a customer engages with the brand.
Decisions are made in real-time based on the most up-to-date customer data and context, allowing for highly responsive and relevant interactions.
Rather than maximizing short-term metrics, it aims to optimize for long-term customer value and overall business outcomes.
This approach differs significantly from the more common “Next Best Action” strategies. While “Next Best Action” typically focuses on selecting the best action from a predefined set of options for a given customer segment, “Next Best Everything” is more comprehensive and flexible. It considers all possible actions across all channels and touchpoints, making decisions at the individual customer level.
The role of reinforcement learning in enabling this approach is crucial. Reinforcement learning algorithms can handle the complexity of individual-level decision-making across multiple touchpoints and over extended time horizons. They learn from each interaction, continuously improving their ability to predict which actions will lead to the best outcomes for each unique customer.
Creating a holistic view of the customer journey is essential for the “Next Best Everything” approach. This involves mapping out all potential touchpoints and interactions a customer might have with your brand, from initial awareness through to long-term loyalty. This journey map serves as the “environment” in which the reinforcement learning agent operates, allowing it to understand the context of each decision and its potential long-term implications.
Implementing a “Next Best Everything” approach using reinforcement learning involves several key steps, each crucial to the success of the system:
This model should encompass all relevant aspects of the customer journey, including customer attributes (demographic information, preferences, purchase history), interaction channels (website, mobile app, email, social media, in-store, customer service), possible actions (product recommendations, promotional offers, content suggestions, service interventions), and external factors (market conditions, competitor actions, seasonal trends). This environment model serves as the playground in which the reinforcement learning agent operates, learning to navigate the complex landscape of customer interactions.
This is perhaps the most critical and challenging aspect of implementing “Next Best Everything.” This function needs to align with your overall business objectives while also capturing the nuances of customer value. It might include components such as short-term revenue, customer satisfaction scores, predicted lifetime value, brand engagement metrics, and retention probability. The key is to create a reward function that encourages the AI to make decisions that benefit both the customer and the business in the long term.
The reinforcement learning agent needs to continually explore new strategies (trying different actions to learn their outcomes) while also exploiting known successful strategies. This balance ensures the system continues to adapt to changing customer preferences and market conditions while still delivering consistent results.
Iterative improvements are fundamental to the success of a “Next Best Everything” approach. The system should be designed to continuously update its models based on new data and outcomes. This might involve regular retraining of the underlying machine learning models, A/B testing of new strategies, and ongoing monitoring and adjustment of the reward function.
The potential benefits of a “Next Best Everything” approach are huge:
Improved customer experience is perhaps the most immediate and noticeable benefit. By tailoring every interaction to the individual customer’s needs and preferences, you can create more relevant, engaging experiences that drive satisfaction and loyalty. This level of personalization can lead to stronger emotional connections between customers and your brand, increasing the likelihood of repeat business and positive word-of-mouth.
Increased customer lifetime value is another benefit. By optimizing for long-term outcomes rather than short-term metrics, you can nurture relationships that generate more value over time. This approach allows you to identify and prioritize high-potential customers early in their lifecycle, investing resources where they’re likely to yield the greatest returns.
“Next Best Everything” also leads to operational efficiencies. Automating complex decision-making across channels can lead to significant time and resource savings. Marketing teams can focus on strategy and creativity, while the AI system handles the intricacies of individual customer interactions.
Better marketing ROI is a natural result of more informed, data-driven decisions about where and how to engage customers. By reducing wasted spend on irrelevant or poorly timed interactions, and focusing resources on the most promising opportunities, you can significantly improve the overall return on your marketing investments.
However, implementing “Next Best Everything” also comes with several challenges that organizations must be prepared to address:
Data requirements are perhaps the most significant hurdle. This approach requires vast amounts of high-quality, real-time data from across the organization. Ensuring data accuracy, completeness, and accessibility can be a major undertaking, especially for companies with legacy systems or siloed data structures.
Technical complexity is another challenge. Implementing reinforcement learning at this scale is a complex task requiring specialized expertise. Many organizations may need to invest in new talent or partnerships to successfully develop and maintain such a system.
Organizational alignment is crucial for success. “Next Best Everything” requires breaking down silos between departments and aligning around a unified customer strategy. This can be a cultural shift for many organizations, requiring strong leadership and change management.
Ethical considerations must also be at the forefront. With the power to make automated decisions that significantly impact customer experiences, it’s crucial to implement safeguards to ensure the AI is making ethical decisions and respecting customer privacy.
As we look to the future, it’s clear that approaches like “Next Best Everything” will play an increasingly important role in customer lifecycle management. To prepare for this shift, organizations should start by assessing their readiness. This involves evaluating current data infrastructure, technical capabilities, and organizational culture.
Key questions to consider include:
Starting with pilot projects can be an effective way to begin the journey towards “Next Best Everything.” This might involve implementing reinforcement learning in a specific channel or for a particular customer segment, allowing you to learn and refine your approach before scaling up.
As we move into this new era of customer lifecycle management, those who embrace these advanced approaches will be well-positioned to lead in their industries. When you’re ready to test the “Next Best Everything” waters, Aqfer is here to help. Our flexible marketing data platform lays a strong foundation for your data-powered marketing offers. Click here to explore our use cases and reach out to get the conversation started.