×
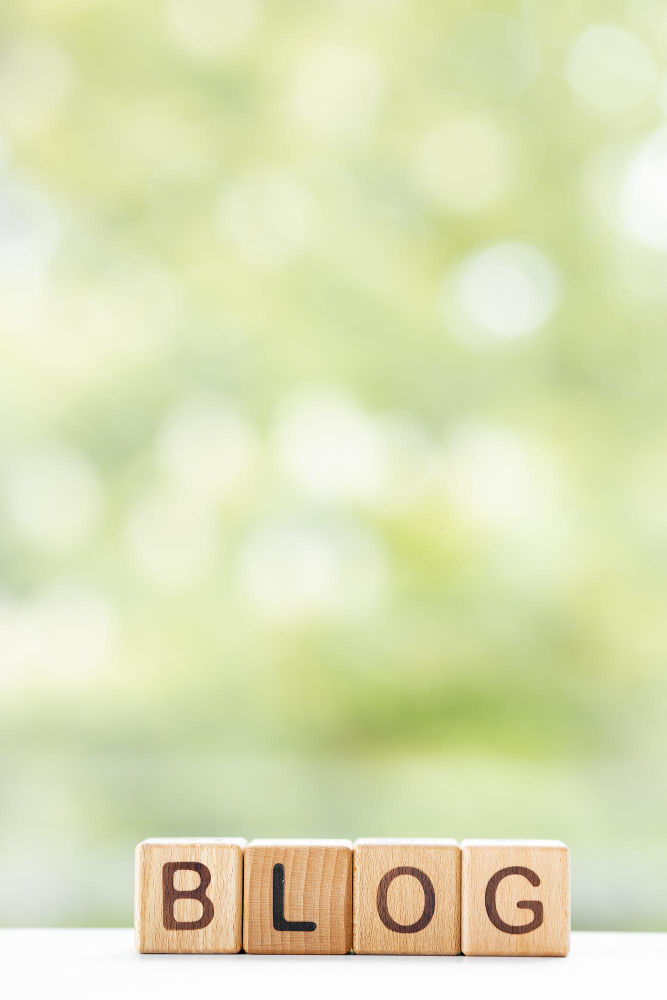
×
Aqfer Insights
Stay on top of the latest trends in Martech, Adtech, and Beyond
Subscribe to follow the news on what’s happening in the marketing data ecosystem.
It’s all the rage to announce how your company is (going to be) delivering on the promise of artificial intelligence (AI). I talk to lots of MADtech providers who know they’ll soon need to better leverage AI and machine learning technologies for advanced analytics, media planning, activation, attribution and more. Many already include the topic prominently on their websites and in their materials.
But, first things first!
Big data technology investments, to date, have typically been underwhelming. AI, if nothing else, will be the next (very) big data technology investment. Some are disillusioned with the outcomes from those investments because they haven’t realized all of the wins they were promised. I personally believe that one of the big wins of generative AI is to make insights more accessible. Being AI ready will mean that as you improve accessibility, exploration results will be quick and extremely cost-effective.
Do a search on “becoming AI ready” and you’ll uncover hundreds of articles talking about people, processes, and platforms. And of course, quality datasets are critical: biases and gaps in data all inform the AI and get over-amplified by AI, leading to garbage in and landfill out. All of those are important.
It is inevitable that this cost will be passed on to the end user.
But there’s a critically under-attended element I want to spotlight here: the cost of cloud computing and its impact on an organization’s ability to leverage AI. According to the linked article, industry estimates peg the cost of running generative AI large language models at up to $4 million a day. Sid Nag, vice president analyst at Gartner says “it is inevitable that this cost will be passed on to the end user.”
There are major considerations around accelerating costs that need to be thought through as AI & ML processes take center stage. As many of us focus on the topic of sustainability, automated big data processes like these can be equivalent to leaving the tap running when you brush your teeth or leaving the lights on all day.
Cloud spend is soaring, but much of it goes to waste, and this waste costs companies a heck of a lot of money. Artificial intelligence is dependent on high quality, organized data to feed the large language models driving the intelligence. It’s not a stretch to imagine where many companies will miss the boat on AI because they didn’t factor into their analysis just how expensive their cloud computing costs would be. Recently, I’ve seen a wave of conversations around sustainability and carbon neutrality within MADtech, which could mean a delay in Artificial Intelligence truly transforming our space.
I was reading this insightful post from Ampersand CEO Nicolle Pangis recently, discussing sustainability as more and more turn their attention to building AI models with correlating increases in data volumes. It got me to thinking that as we move into a new world around artificial intelligence, are companies aware of what is going to happen to their cloud computing costs? And in general, have they thought through the waste in compute costs that can be avoided by having a sound and proven approach to data and technology driving large language models?
I’m highlighting a critical step that many are missing: the ingestion, cleaning and organization of very large and often disparate data sets. Keep in mind, the degree to which AI large language models can process and make sense of the data that feeds them is largely a function of how well those data sets are prepared. I’d be remiss if I didn’t mention that my company, Aqfer, has optimized its data organization and collation processes to be best-in-class: we recently ran a benchmark study that showed we drive a 16x improvement, relative to typical Spark-based solutions, in the speed to collate data, leading to substantial cost savings on cloud computing expenses.
Cloud computing provides the system resources and infrastructure needed to train and deploy AI models at scale. Cost control is imperative. We think this is a foundational, cost-effective step to begin the AI journey.
Aqfer’s Marketing Data Platform as a Service (MDPaaS) is a robust solution that enables companies to become “AI Ready.” It provides a comprehensive Lakehouse that integrates advertising, marketing, prospect, and customer data from various commercial and enterprise data sources. Data is managed in a next-generation Serverless LakeHouse, designed to handle large volumes of digital advertising and marketing data in its most granular form. The platform’s flexibility allows for customization at each layer in the solution, including the Data, Services, and Application Layers. This means businesses can efficiently and cost-effectively build and deploy applications that leverage customer profile and interaction data for marketing and advertising purposes. By providing a unified view of high quality, organized data, Aqfer’s platform empowers companies to leverage AI and machine learning technologies for advanced analytics, media planning, attribution, activation, audience analysis, and more.